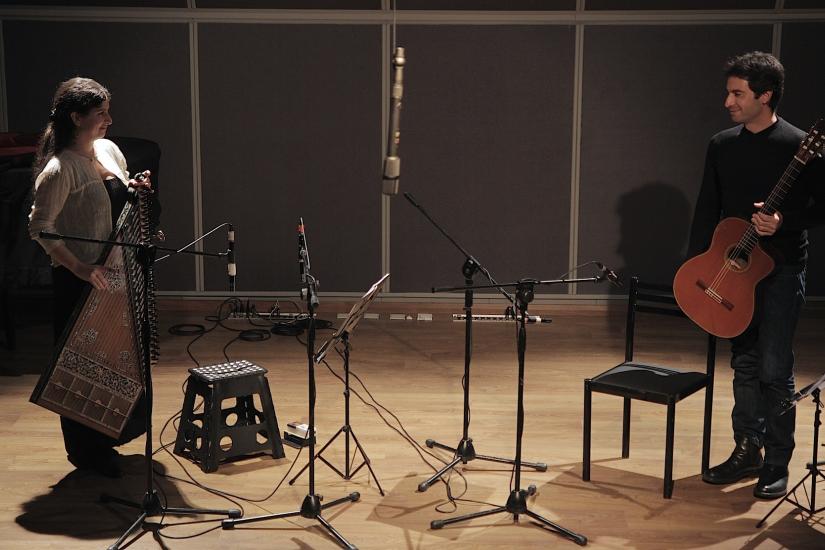
ABSTRACT | Jaynes’s maximum entropy method provides a family of principled models that allow the prediction of a system’s properties as constrained by empirical data (observables), which in sociology are also known as p* models. However, their use is often hindered by the degeneracy problem characterized by spontaneous symmetry breaking, where predictions and thus the modeling fail. Here we show that degeneracy appears when the corresponding density of states function is not log-concave, which is the consequence of nonlinear relationships between the constraining observables. We illustrate this phenomenon on several examples, including complex networks, combinatorics, classical spin systems, and horse-race predictions. Exploiting these nonlinear relationships we then propose a solution to the degeneracy problem for a large class of systems via non-linear transformations that render the density of states function log-concave. The effectiveness of the method is demonstrated on real-world network data. Finally, we discuss the implications of these findings on a possible, purely geometrical formulation of phase transitions, showing that they are a mathematical phenomenon, more general than what we have learned from their standard physics interpretation.
BIO | Zoltan Toroczkai is a Professor of Physics and Concurrent Professor of Computer Science and Engineering at the University of Notre Dame. After receiving a PhD in theoretical physics from Virginia Tech, he spent his postdoctoral years at the University of Maryland, then at Los Alamos National Laboratory (LANL) as Director Funded Fellow. He then joined the research staff at LANL and became the Deputy Director of the Center for Nonlinear Studies at LANL, until moving to the University of Notre Dame in 2006. His research is in the areas of statistical physics and mathematical physics on topics including fluids, reaction kinetics, population dynamics, agent-based systems, game theory, complex networks, foundations of computing and brain neuronal networks. In November 2012, he was elected APS Fellow “for his contributions to the understanding of the statistical physics of complex systems, and in particular for his discoveries pertaining to the structure and dynamics of complex networks”.