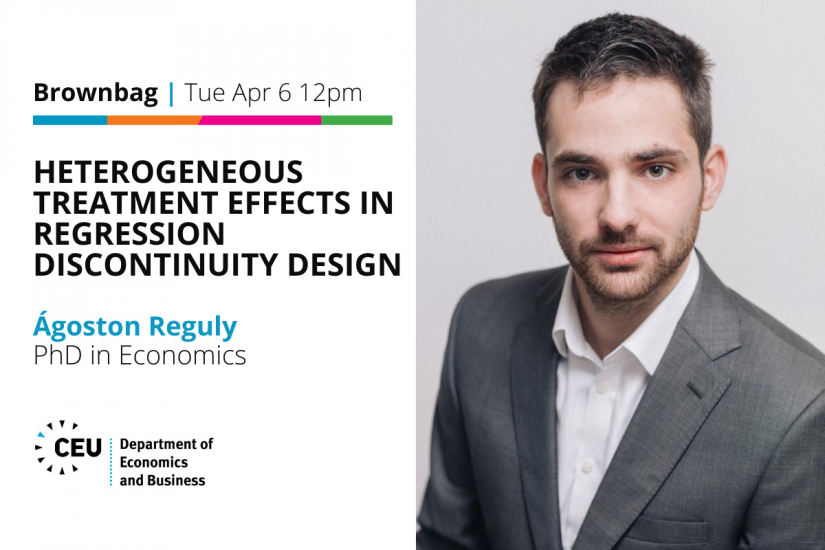
Online Event
Abstract
This paper constructs an algorithm, which uncover treatment effect heterogeneity in classical regression discontinuity (RD) design. I propose an algorithm: honest regression discontinuity tree to identify heterogeneity in the treatment effect across sub-populations using additional features or covariates. The relevant sub-populations are a prior unknown, and it is the task of the algorithm to discover them, without invalidating inference.
The paper contributes to two literature: for the regression discontinuity designs with the estimate of conditional average treatment effects (CATE) with potentially many covariates with one running variable and with a known threshold value. For the causal machine learning literature with a new criterion to estimate causal effects, where the effects are estimated by leaf-by-leaf regressions.
To show how the algorithm works in practice, I use Pop-Eleches and Urquiola (2013) data on Romanian school system, and uncover heterogeneous effects on the impact of going to a better school.