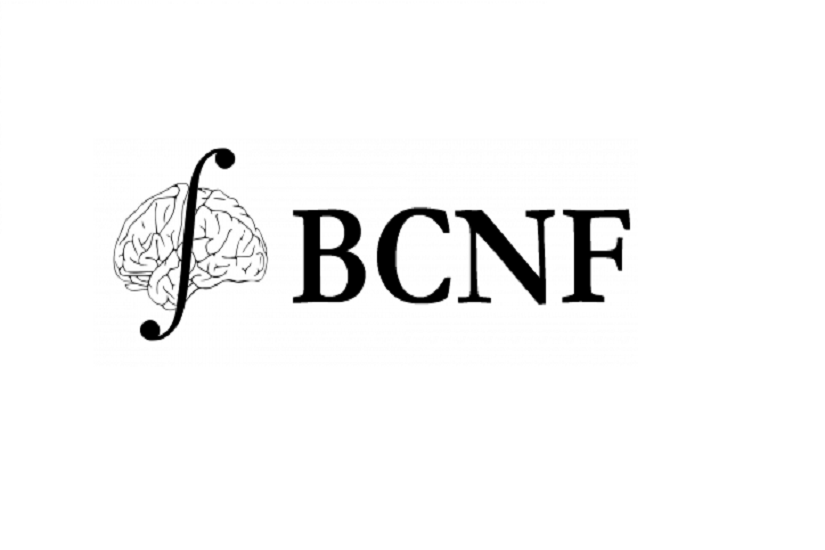
The Budapest Computational Neuroscience Forum is a series of informal monthly meetings of Budapest-based computational neuroscientists and computational cognitive scientists with the aim of facilitating discussion and cooperation among researchers working in different institutes and giving an opportunity to students to present their work and get to know the comunity. Originally started in 2007, restarted in 2017 and then again in 2023 the Forum is now regularly hosted by Central European University, and followed by a social event, both open to anyone interested.
Events of the Forum are advertised on a mailing list. If you wish to be on this list or have any inquiries about the series, contact Mihály Bányai.
Upcoming meeting:
Time: 17:00, October 25, 2023.
Location: CEU, 1051 Bp. Nádor u. 15, Room FT. 408 (restricted entry, guest cards will be available at the reception)
Speaker: Ferenc Csikor, Wigner Institute
Title: Top-down perceptual inference shaping the activity of early visual cortex
Abstract: Deep discriminative models have recently provided remarkable insights into hierarchical processing in the brain by predicting neural activity along the visual ventral pathway. However, these models are at odds with biological systems both at the computational and architectural levels: on the computational level, deep discriminative models rely on supervised learning, which necessitates exhaustive labeling of experiences; and on architectural level, these models are fundamentally feed-forward in processing incoming stimuli, in contrast with the ventral pathway that is characterized by extensive top-down connectivity. Here, we address these issues by developing a hierarchical deep generative model of natural images and show that it can predict an extensive set of experimental results in the primary and secondary visual cortices (V1 and V2, respectively). Our analysis shows that sensitivity of V2 neurons to subtle changes of high-level statistics of images is a consequence of learning a hierarchical representation of natural images. Further, we show that top-down influences are natural ingredients of hierarchical generative models, and a range of experimental phenomena concerning the mean responses and noise correlation structure of V1 responses are a consequence of inference in this generative model.